Today I encountered a lot of OpenCV loading model errors when debugging the yolov7 model conversion and loading problemm There is no way to fully display it due to the title length limit, I will post it here in its entirety.
[ERROR:0] global D:\opencv-python\opencv\modules\dnn\src\onnx\onnx_importer.cpp (720) cv::dnn::dnn4_v20211004: :ONNXImporter::handleNode DNN/ONNX: ERROR during processing node with 5 inputs and 1 outputs: [NonMaxSuppression]:(onnx::Gather_384)
cv2.error: OpenCV(4.5.4) D:\opencv-python\opencv\modules\dnn\src\onnx\onnx_importer.cpp:739: error: (- 2:Unspecified error) in function 'cv::dnn::dnn4_v20211004::ONNXImporter::handleNode'
cv2.error: OpenCV(4.5.4) D:\opencv-python\opencv\modules\dnn\src\onnx\onnx_importer.cpp:739: error: (- 2:Unspecified error) in function 'cv::dnn::dnn4_v20211004::ONNXImporter::handleNode'
> Node [NonMaxSuppression]:(onnx::Gather_384) parse error: OpenCV(4.5.4) D:\opencv-python\opencv\modules\dnn\src\dnn.cpp:615: error: (-2:Unspecified error) Can't create layer "onnx::Gather_384" of type "NonMaxSuppression" in function 'cv::dnn::dnn4_v20211004::LayerData::getLayerInstance&# 39;
At this time, I think of a way to compare my own model with the official model one by one,Comparison of one node and one node, Finally found the problem at the end.
[Official Model]
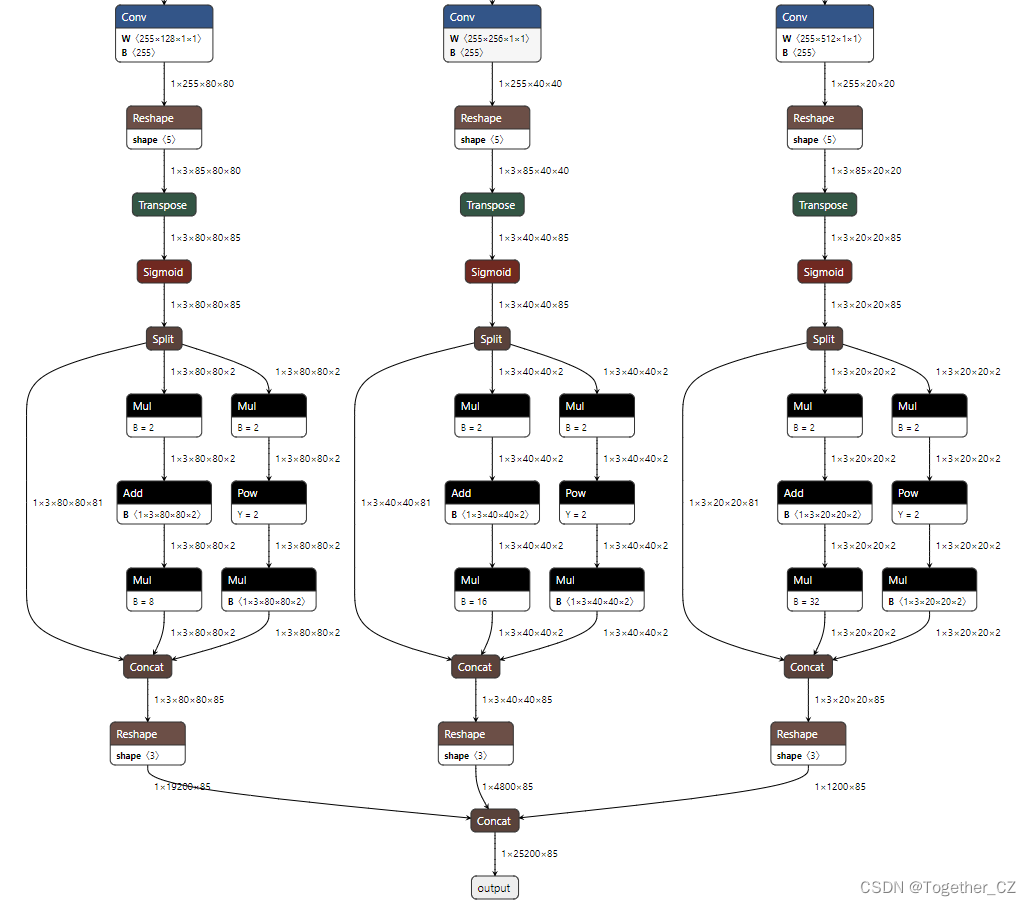
[My own model]
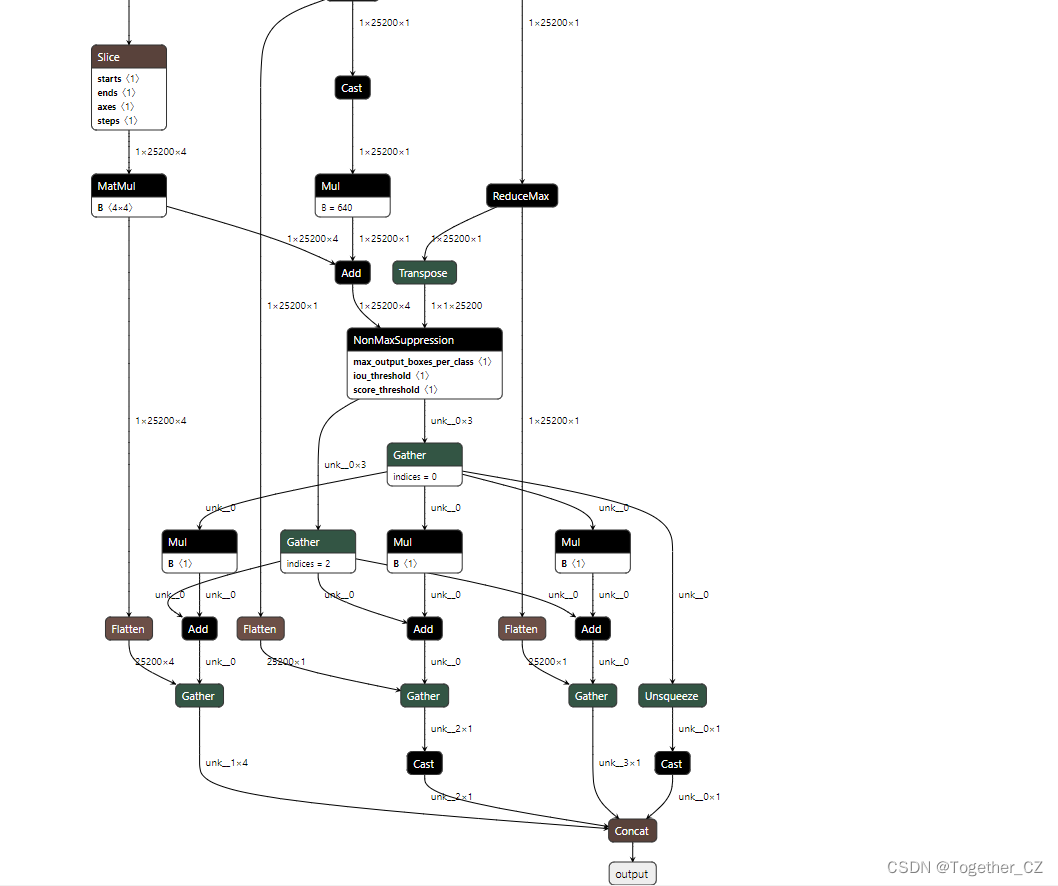
Seeing this, I’m wondering if there is such a big difference??It shouldn’t be,It’s all models built from the same code,So I started to trace the source,Sure enough Problem found.
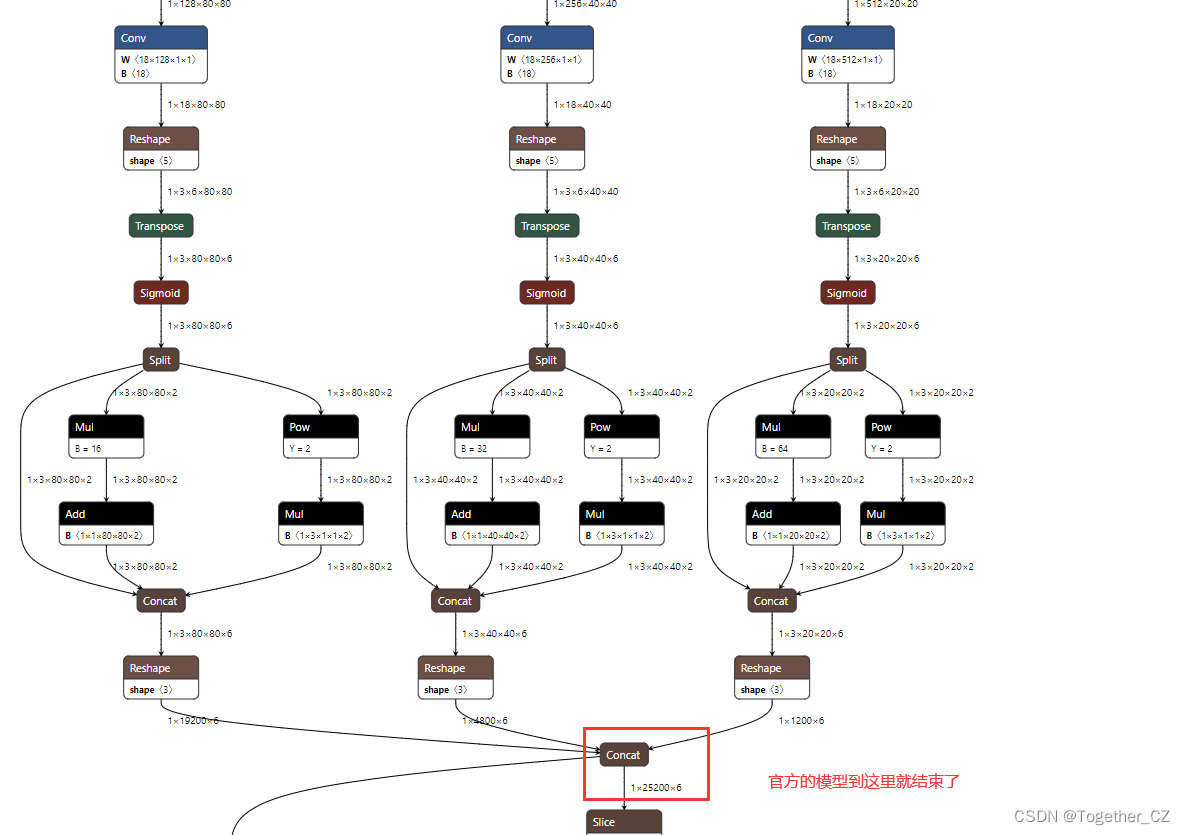
At the position of my red frame, the official model ends here, and there is a large string of, tensor shapes for debugging both by printing, I guess that there may be a problem with the parameter settings during the model export process, So I tried to verify basically all the uncertain parameters, I found the problem.
In order to facilitate your understanding, I am giving my original conversion operation command here:
python export.py --weights best.pt --grid --end2end --simplify --topk-all 100 --iou-thres 0.65 --conf-thres 0.35 --img-size 640 640 --max-wh 640
This is the command after:
python38 export.py --weights best.pt --grid --simplify --topk-all 100 --iou-thres 0.65 --conf-thres 0.35 --img -size 640 640 --max-wh 640
See the difference, In fact, it is caused by the parameter end2end, After the modification, my model is as follows:
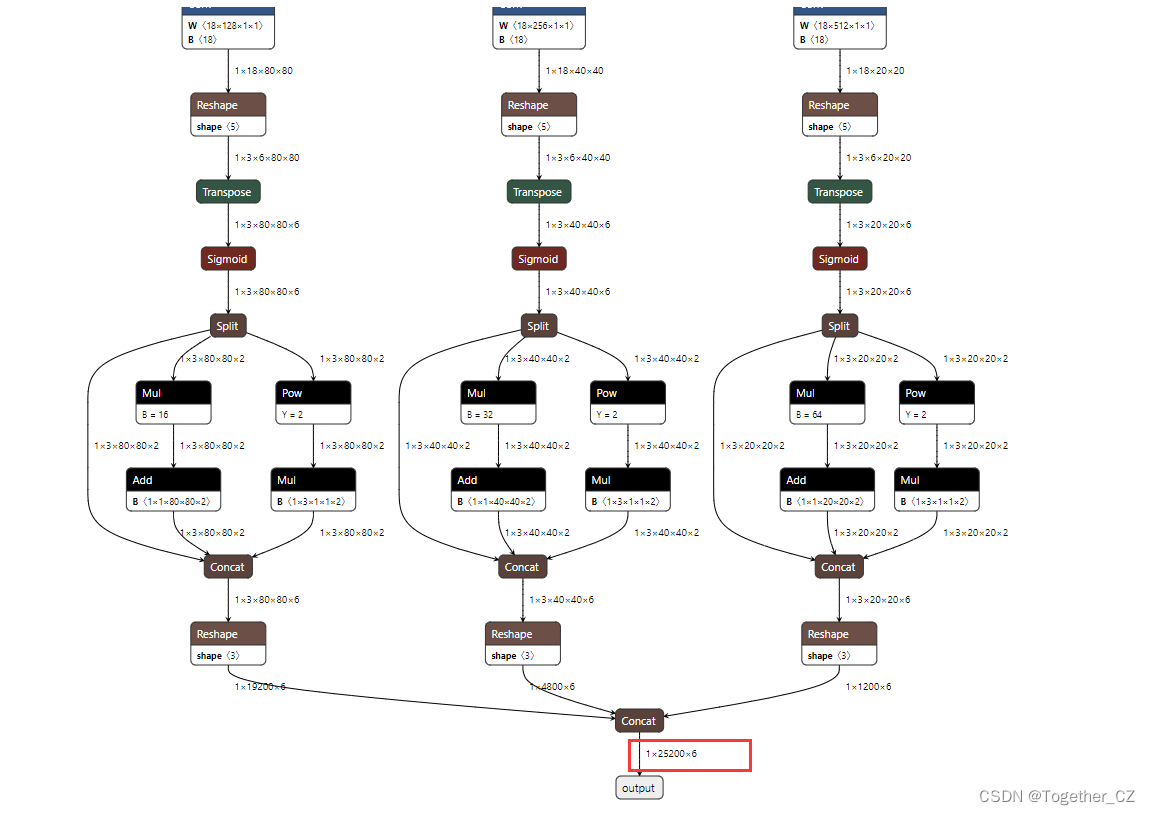
Because what I am doing here is the detection of the category, so the final output is: 1x25200x6, and the official one is: 1x25200x85.