· Introduction of the evaluation index EER(Equal Error Rate)
In-depth learning articles generally use Error probabilities such as EER(Equal Error Rate) as an objective criterion for measuring classifiers, and the RECEIVER Operating Characteristic (ROC) curve explains how to calculate EER.
Here is a brief introduction to the EER calculation
EER (Average error probability) is a biometric security system algorithm used to pre-determine its error acceptance rate and its error rejection rate threshold. When the rates are equal, the common value is called the equal error rate. This value indicates that the proportion of false acceptances is equal to the proportion of false rejections. The lower the iso-error rate, the higher the accuracy of biometric identification system.
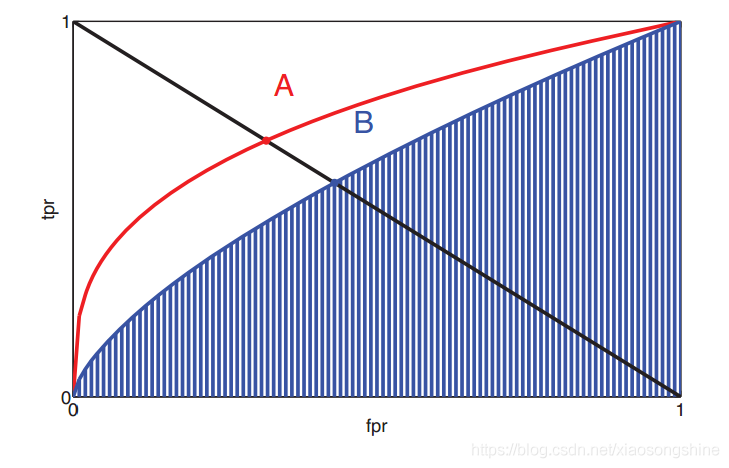
Use other ROC evaluation criteria
AUC (area under thecurve), also is the area under the ROC curve, the greater the classifier, the better, the maximum value is 1, the blue stripes in the graph area is the blue curve corresponding AUCEER (equal error rate), that is, the value of the FPR = FNR, due to the FNR = 1 – TPR, can draw A from (0, 1) to (1, 0) in A straight line, finding the intersection point, in the figure A and B two points.
In-depth learning articles generally use Error probabilities such as EER(Equal Error Rate) as an objective criterion for measuring classifiers, and the RECEIVER Operating Characteristic (ROC) curve explains how to calculate EER.
Here is a brief introduction to the EER calculation
EER (Average error probability) is a biometric security system algorithm used to pre-determine its error acceptance rate and its error rejection rate threshold. When the rates are equal, the common value is called the equal error rate. This value indicates that the proportion of false acceptances is equal to the proportion of false rejections. The lower the iso-error rate, the higher the accuracy of biometric identification system.
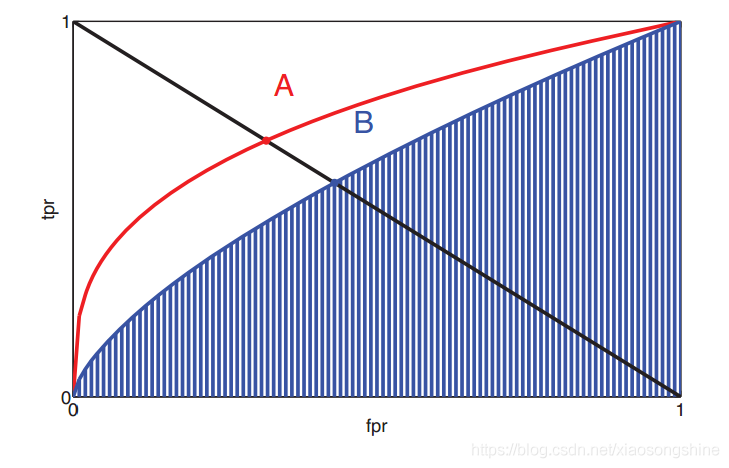
Use other ROC evaluation criteria
AUC (area under thecurve), also is the area under the ROC curve, the greater the classifier, the better, the maximum value is 1, the blue stripes in the graph area is the blue curve corresponding AUCEER (equal error rate), that is, the value of the FPR = FNR, due to the FNR = 1 – TPR, can draw A from (0, 1) to (1, 0) in A straight line, finding the intersection point, in the figure A and B two points.
Read More:
- The difference between single equal sign and double equal sign EQ in shell script
- Introduction to JIRA introduction to HP ALM
- Token bucket implementation with adaptive token generation rate
- Must have equal len keys and value when setting with an Iterable
- Info:Memory module [DIMM] needs attention: Single-bit warning error rate exceeded, Single-bit fai…
- Markdown and latex: approximately equal to the writing of symbols
- Driver IRQL Not Less or Equal (rtwlane.sys) blue screen restart problem win8.1
- Introduction to VTK
- Brief introduction of Linux MMAP and solution of bus error
- pthread_ Introduction and application of join function
- Introduction to total phase data center
- Brief introduction of idea Lombok and solutions for reporting red and wrong
- Introduction of Hadoop HDFS and the use of basic client commands
- GoogleIO 2013 Android fast networking framework Volley introduction
- Introduction to the principle of Mali tile based rendering
- Wrong classification of Python — assertion cur_ Target is greater than or equal to 0 and cur_ Target is less than n_ classes failed
- Brief introduction of cmake generating all in vs Project_ BUILD、INSTALL、ZERO_ Check function!!
- How to solve the problem of error reporting in the introduction of Python Django no module name ‘Django. Utils. Six’
- Java — one of Apollo configuration centers — Introduction to Apollo
- The introduction of third-party jar package in mavn project results in classnotfoundexception