
abstract
Many cultures in the world believe that palm reading can be used to predict a person’s future life. Palmology uses features of the hand, such as the lines of the palm, the shape of the hand, or the position of the fingertips. However, the research of palmprint detection is still relatively small, many people use the traditional image processing technology. In most real scenes, the image is usually not very good, which leads to a serious lack of performance of these methods. This paper presents an algorithm to extract main palmprint from palm image. Our method uses deep learning networks (DNNS) to improve the performance. Another challenge to this problem is the lack of training data. In order to solve this problem, the author made a data set from scratch. From this data set, the author compares the performance of the existing method with that of the author’s method. In addition, based on the structure of UNET segmentation Neural Network and the knowledge of attention mechanism, an efficient palmprint detection structure is proposed. In order to improve the segmentation accuracy, a context fusion module is proposed to capture the most important context features. The experimental results show that the F1 score of this method is the highest, about 99.42%, and the Miou is 0.584 on the same data set, which is better than other methods.

Innovation of the paper
To sum up, the main contributions of the author are summarized as follows:
DNN is proposed to solve the problem of palmprint detection. The author uses deep learning and image processing technology instead of pure traditional image processing as in other previous papers. Provide high quality data set for this problem. The data set was carefully annotated by professionals in the field. The model and context fusion module proposed by the author can achieve high accuracy even in complex palmprint images. Through this method, the author has achieved good results in Miou score.

Frame structure

The author’s image segmentation system architecture
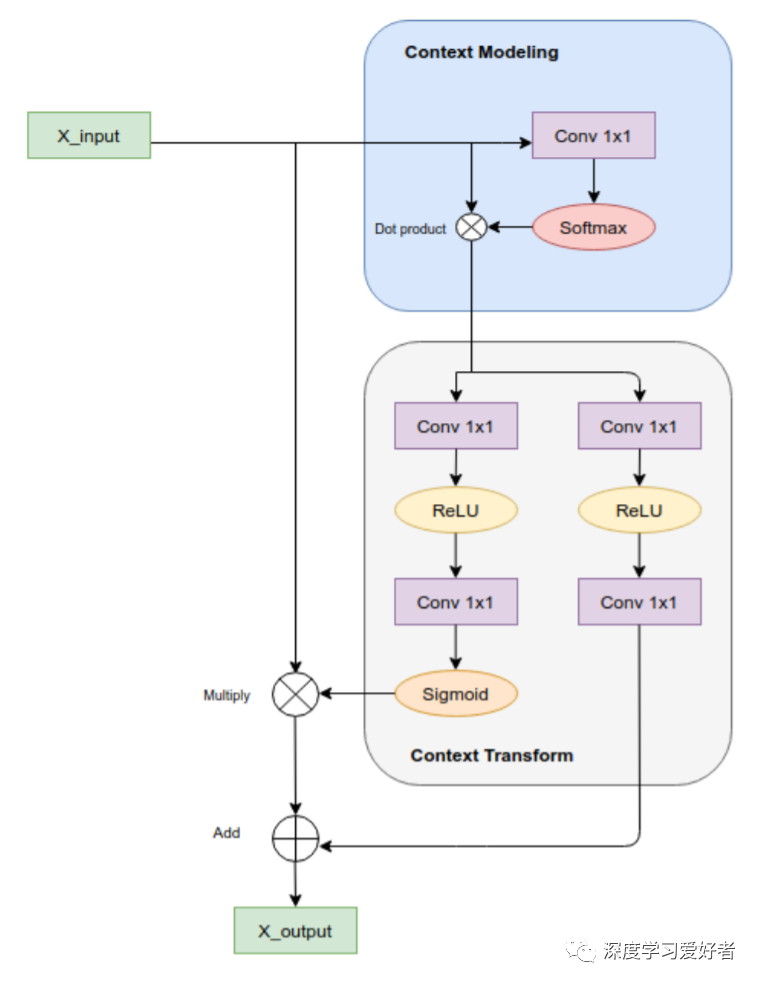
Context fusion module integrating local and global context features
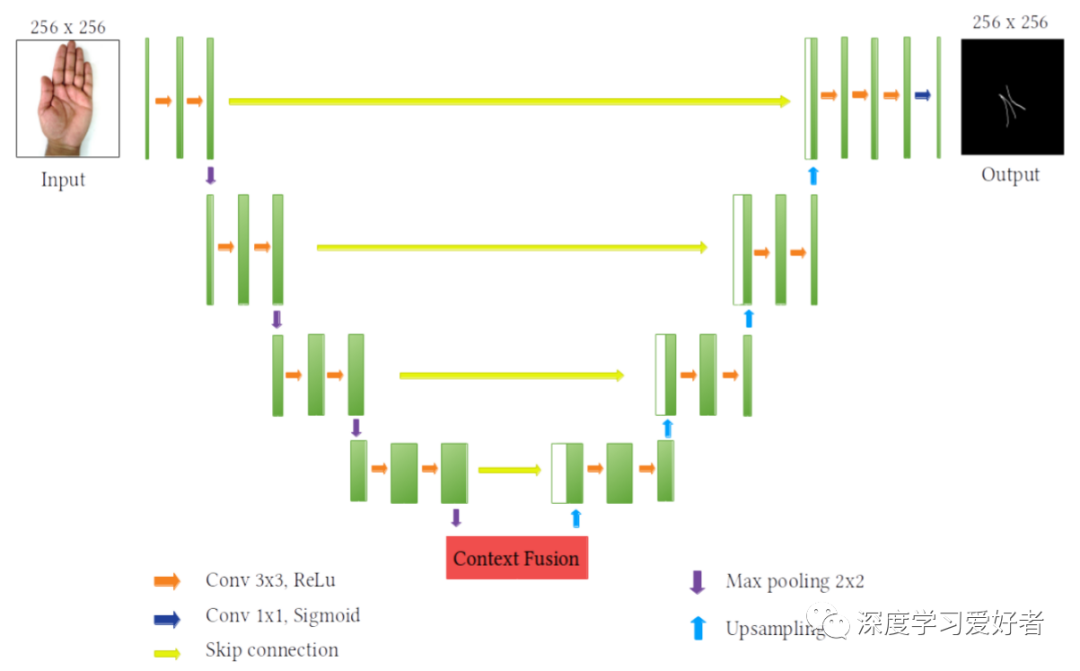
U-net has context fusion module

experimental result
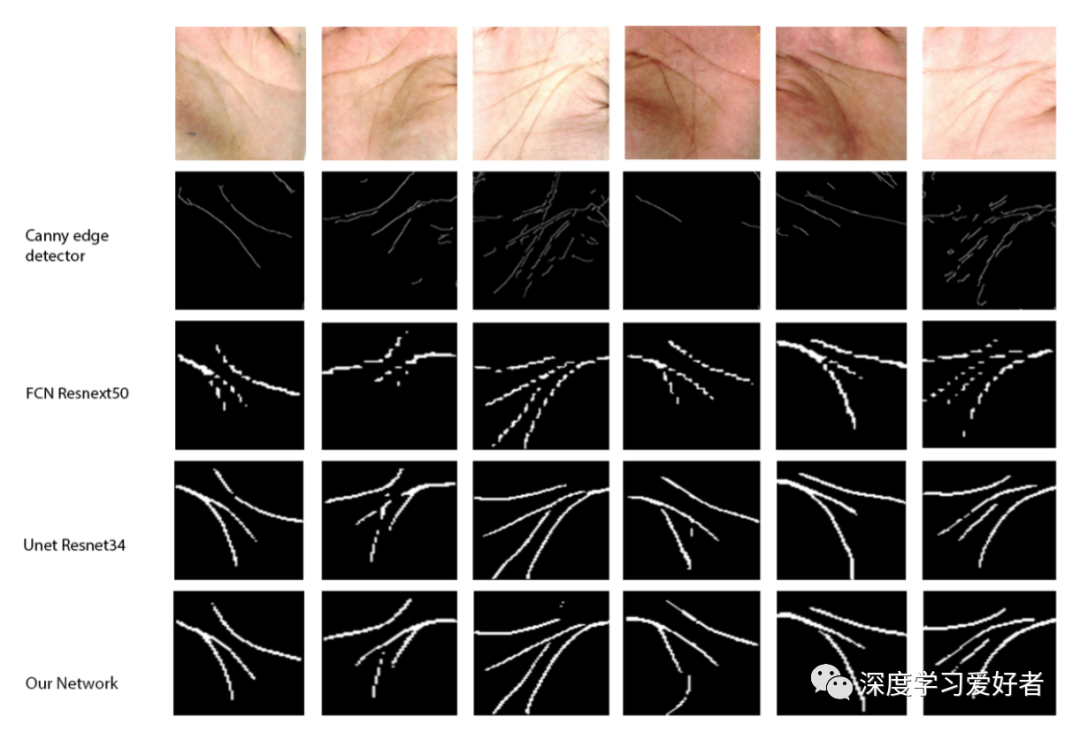
The output images of some models are applied to this problem. The author’s network (UNET CFM) has achieved good results in complex palmprint input.
Conclusion 
In this paper, the author uses deep learning technology to establish neural network to solve the problem of palmprint segmentation. In the author’s data set, the final Miou of the author’s model is 0.584, and the F1 score is 99.42%. The data set is manually collected and will be published for scientific purposes. Experimental results show that this method has great advantages over traditional image processing in palmprint image segmentation task. The future work of this study will be to study a more robust method to deal with the changes of complex background images; in addition, other functions of CFM can be used for further investigation.
Link to the paper: https://arxiv.org/pdf/2102.12127.pdf
Original text: https://mp.weixin.qq.com/s/T_ kIP8F-usNfZBzRTIk0jA